PyPy and conda-forge
You can use PyPy as your python interpreter in a conda environment. The conda-forge team has graciously provided this service.
The conda-forge tips-and-tricks page says:
The conda-forge channel supports creating and installing packages into environments using the PyPy interpreter. Many packages are already available. You need to enable the conda-forge channel and use the pypy identifier when creating your environment:
$ conda create -c conda-forge -n my-pypy-env pypy python=3.8 $ conda activate my-pypy-env
Currently supported python versions are 3.8 and 3.9. Support for pypy3.7 has been dropped. While you can still create a python 3.7 environment, you you will not be getting updates as new package versions are released (including pypy itself).
if you are using defaults as a low priority channel, then you need to use strict channel priority as the metadata in defaults has not been patched yet which allows cpython extension packages to be installed alongside pypy.
$ conda config --set channel_priority strict
The work required some out-of-the-box thinking on the part of conda-forge since
they needed to add the idea of a pypy
identifier to the python version and
the whole conda team has been very supportive of the effort needed. Binary
packages are on offer for the usual platforms:
-
x86_64
windows, macos, linux -
ppc64le
andaarch64
linux.
There are currently over 1000 packages available for download via the conda-forge channel, and more are being added as the kind package maintainers work around various differences between CPython and PyPy. Please let us know if your favorite package is not supported.
The PyPy Blog Turns 15 Years
Exactly 15 years ago today we wrote the first blog post on the PyPy blog! Over the years, we have written 423 posts, from the shortest to the longest. In 2021 we moved from blogger to our own domain.
The topics over the years varied widely, we published release announcements; roadmaps; JIT, GC and STM updates; benchmarks; sprint, trip and conference reports; technical deep dives; case studies; april fool's jokes; research projects; other languages using RPython; finished PhD Bachelor and Master, theses; pictures:
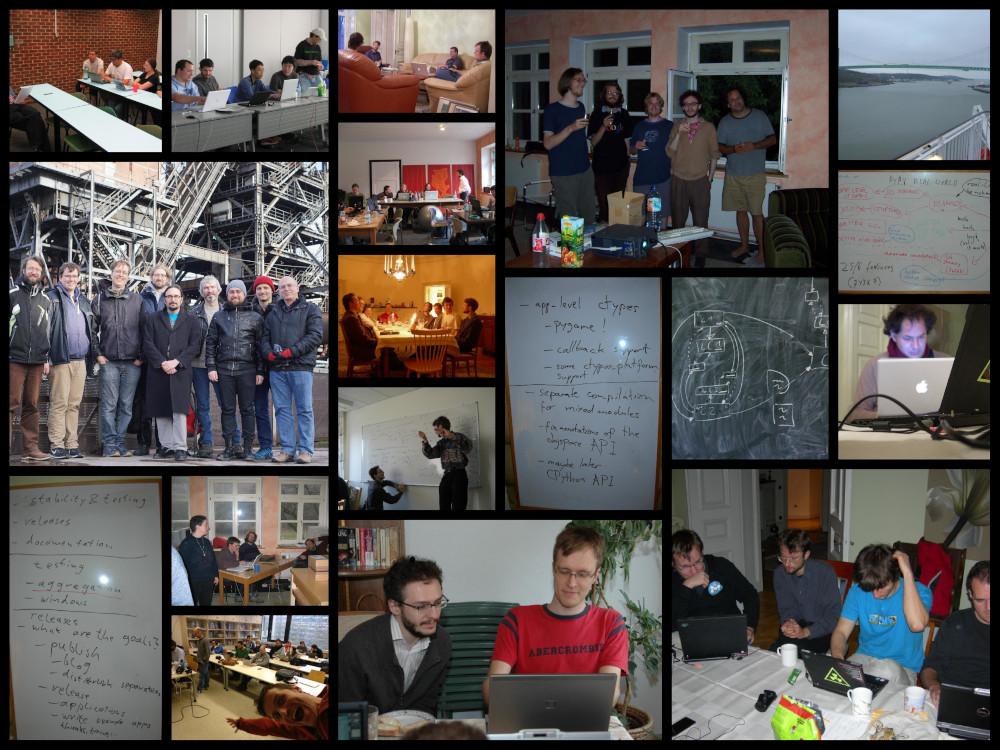
and diagrams:
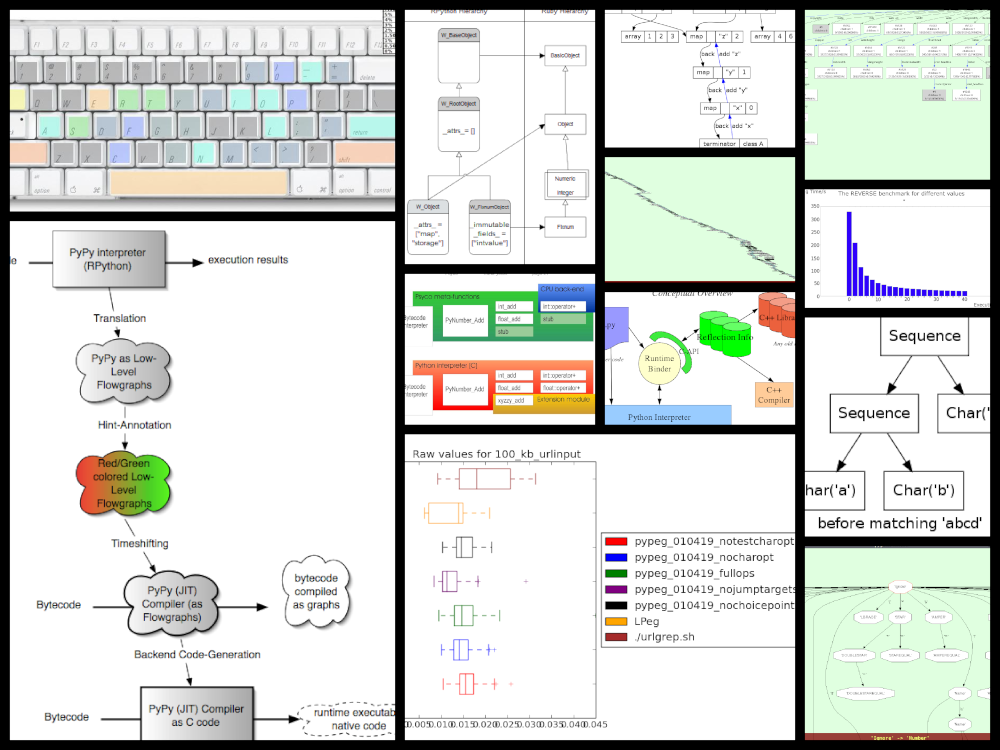
Quite a number of blog posts were very early iterations of papers that we published later, here are a few that I can remember:
Applying a Tracing JIT to an Interpreter became Tracing the meta-level: PyPy's tracing JIT compiler at ICOOOLPS 2009, by far our most successful paper.
Escape Analysis in PyPy's JIT became Allocation removal by partial evaluation in a tracing JIT at PEPM 2010.
Controlling the Tracing of an Interpreter With Hints was a draft of the paper Runtime feedback in a meta-tracing JIT for efficient dynamic languages at ICOOOLPS 2011
Using Escape Analysis Across Loop Boundaries for Specialization was the nucleus of Loop-aware optimizations in PyPy's tracing JIT at DLS 2012.
List Strategies was eventually turned into the paper Storage strategies for collections in dynamically typed languages at OOPSLA 2013.
Greatest Hits
In terms of visitors, the top five posts on the old blog were – on the new blog we simply don't have stats (yet?):
The number of posts per year developed like this:
The most prolific authors are:
Several blog posts have made it to the Hacker News front page, three of them to number 1:
Personal Favourites
While looking through the posts, there were a few that stood out to me in some way, so here's a subjective list of ones that I had fun looking at again:
2009: PyPy gets a new compiler
2012: Architecture of Cppyy
2013: 10 years of PyPy
2014: PyPy IO Improvements
2016: PyPy Enterprise Edition
We'd like to thank our authors, guest authors, commenters, users and readers who have stuck with us through one and a half decades! If there's any particular topics you would like to read something about, or any guest posts you'd like to write, let us know!
Allocation Removal in the Toy Optimizer
One of the workhorse optimization of RPython's tracing JIT is allocation removal, which removes short-lived object allocation from traces. Many Python programs create a lot of objects that only live for a short time, and whose lifespan is fully predictable (common examples are integer and float boxes, but also tuples, frames, intermediate string results, etc). Allocation removal will try (and very often succeed) to remove these allocations from traces. In this blog post I want to show a toy version of how allocation removal is implemented.
In the previous blog post of this series I showed the complete code for writing a toy one-pass optimizer that does constant folding, common subexpression elimination and strength reduction. In this second post, I want to use allocation removal as a more advanced optimization pass. The basic optimization framework is the same, we will use the same datastructures for intermediate representation and also keep using the same union find data structure to store equivalences between IR operations. Here's the infrastructure code from the last post:
import pytest from typing import Optional, Any class Value: def find(self): raise NotImplementedError("abstract") def _set_forwarded(self, value): raise NotImplementedError("abstract") class Operation(Value): def __init__( self, name: str, args: list[Value] ): self.name = name self.args = args self.forwarded = None self.info = None def __repr__(self): return ( f"Operation({self.name}, " f"{self.args}, {self.forwarded}, " f"{self.info})" ) def find(self) -> Value: op = self while isinstance(op, Operation): next = op.forwarded if next is None: return op op = next return op def arg(self, index): return self.args[index].find() def make_equal_to(self, value: Value): self.find()._set_forwarded(value) def _set_forwarded(self, value: Value): self.forwarded = value class Constant(Value): def __init__(self, value: Any): self.value = value def __repr__(self): return f"Constant({self.value})" def find(self): return self def _set_forwarded(self, value: Value): assert ( isinstance(value, Constant) and value.value == self.value ) class Block(list): def opbuilder(opname): def wraparg(arg): if not isinstance(arg, Value): arg = Constant(arg) return arg def build(self, *args): # construct an Operation, wrap the # arguments in Constants if necessary op = Operation(opname, [wraparg(arg) for arg in args]) # add it to self, the basic block self.append(op) return op return build # a bunch of operations we support add = opbuilder("add") mul = opbuilder("mul") getarg = opbuilder("getarg") dummy = opbuilder("dummy") lshift = opbuilder("lshift") # some new one for this post alloc = opbuilder("alloc") load = opbuilder("load") store = opbuilder("store") print = opbuilder("print") def bb_to_str(bb: Block, varprefix: str = "var"): def arg_to_str(arg: Value): if isinstance(arg, Constant): return str(arg.value) else: return varnames[arg] varnames = {} res = [] for index, op in enumerate(bb): var = f"{varprefix}{index}" varnames[op] = var arguments = ", ".join( arg_to_str(op.arg(i)) for i in range(len(op.args)) ) strop = f"{var} = {op.name}({arguments})" res.append(strop) return "\n".join(res)
There are two changes to the code from the last post: Operation
instances
have a new .info
field, which is set to None
by default. We will learn
how the info field is used a bit further down. Also, we define some new
operations.
Interpreter
In this post we will mainly concern ourselves with optimizing
programs that allocate memory. We assume that our language is garbage collected
and memory safe. The new operations that we will optimize are alloc
(allocates some new object), store
(stores a value into a fixed field of an
object), load
(loads the value from a field in the object).
We are leaving out a lot of details of a "real" system here, usually an
alloc
operation would get some extra information, for example the type of
the freshly allocated object or at least its size. load
and store
would
typically have some kind of field offset and maybe some information about the
field's type
Here's a simple program that uses these operations:
var0 = getarg(0) obj0 = alloc() store(obj0, 0, var0) var1 = load(obj0, 0) print(var1)
The code allocates a new object obj0
, stores var0
into field 0
of
the object, the loads the same field and prints the result of the load.
Before we get started in writing the optimizer for these operations, let's try
to understand the semantics of the new operations a bit better. To do this, we
can sketch a small interpreter for basic blocks, supporting only getarg
,
alloc
, store
, load
, print
:
def test_interpret(): bb = Block() var0 = bb.getarg(0) obj = bb.alloc() sto = bb.store(obj, 0, var0) var1 = bb.load(obj, 0) bb.print(var1) assert interpret(bb, 17) == 17 class Object: def __init__(self): self.contents: dict[int, Any] = {} def store(self, idx : int, value : Any): self.contents[idx] = value def load(self, idx : int): return self.contents[idx] def get_num(op, index=1): assert isinstance(op.arg(index), Constant) return op.arg(index).value def interpret(bb : Block, *args : tuple[Any]): def argval(op, i): arg = op.arg(i) if isinstance(arg, Constant): return arg.value else: assert isinstance(arg, Operation) return arg.info for index, op in enumerate(bb): if op.name == "getarg": res = args[get_num(op, 0)] elif op.name == "alloc": res = Object() elif op.name == "load": fieldnum = get_num(op) res = argval(op, 0).load(fieldnum) elif op.name == "store": obj = argval(op, 0) fieldnum = get_num(op) fieldvalue = argval(op, 2) obj.store(fieldnum, fieldvalue) # no result, only side effect continue elif op.name == "print": res = argval(op, 0) print(res) return res else: raise NotImplementedError( f"{op.name} not supported") op.info = res
The interpreter walks the operations of a block, executing each one in turn. It
uses the info
field to store the result of each already executed
Operation
. In this interpreter sketch we stop at the first print
that
we execute and return its argument for the simple but bad reason that it makes
test_interpret
easier to write.
Objects in the interpreter are represented using a class Object
, which
stores the object's field into a Python dictionary. As written above, this is a
simplification, in a real system the alloc operation might for example take
some kind of type as an argument, that describes which kinds of fields an
object has and how they are laid out in memory, which would allow more
efficient storage of the content. But we don't want to care about this level of
detail in the post, so using a dict in the interpreter is good enough.
Version 1: Naive Attempt
In many programs, some allocated objects don't live for very long and have a completely predictable lifetime. They get allocated, used for a while, and then there is no way to reference them any more, so the garbage collector will reclaim them. The very first example block had such an allocation:
var0 = getarg(0) obj0 = alloc() store(obj0, 0, var0) var1 = load(obj0, 0) print(var1)
Here obj0
is written to, then read from, and then it's no longer used. We
want to optimize such programs to remove this alloc
operation. The optimized
version of this program would look like this:
var0 = getarg(0) print(var0)
The alloc
, store
and load
operations have been completely removed.
This is a pretty important optimizations for PyPy's JIT: Allocations, memory
reads and writes are quite costly and occur a lot in Python, so getting rid
of as many of them as possible is instrumental for performance.
Implementing the optimization is not a lot of code! However, understanding all the corner cases of the optimization and making sure that the resulting program behave correctly is not completely trivial. Therefore we will develop the optimization step by step, in a test driven fashion: I will start each section with a new test that shows a bug in the version of the optimization that we have so far.
Let's start in a really naive way. Here's the first test we would like to pass, using the example program above:
def test_remove_unused_allocation(): bb = Block() var0 = bb.getarg(0) obj = bb.alloc() sto = bb.store(obj, 0, var0) var1 = bb.load(obj, 0) bb.print(var1) opt_bb = optimize_alloc_removal(bb) # the virtual object looks like this: # obj # ┌──────────┐ # │ 0: var0 │ # └──────────┘ assert bb_to_str(opt_bb, "optvar") == """\ optvar0 = getarg(0) optvar1 = print(optvar0)"""
We will define a class VirtualObject
that is basically identical to
Object
above. But it will not be used by the interpreter, instead we will
use it during optimization.
class VirtualObject: def __init__(self): self.contents: dict[int, Value] = {} def store(self, idx, value): self.contents[idx] = value def load(self, idx): return self.contents[idx]
The structure of the optimizer is going to be like those in the first blog post. The optimizer makes a single pass over all operations. It removes some and emits others.
This first version of the allocation removal optimizer is going to be extremely
optimistic. It simply assumes that all the allocations in the program can be
optimized away. That is not realistic in practice. We will have to
refine this approach later, but it's a good way to start. That means whenever
the optimizer sees an alloc
operation, it removes it and creates a
VirtualObject
object which stores the information that is known during
optimization about the result of the alloc
. Like in the interpreter, the
VirtualObject
is stored in the .info
field of the Operation
instance
that represents the alloc
.
When the optimizer sees a store
operation, it will also remove it and
instead execute the store by calling the VirtualObject.store
method.
Here is one important difference between the interpreter and the optimizer: In
the interpreter, the values that were stored into an Object
(and thus
put into the object's .contents
dictionary) were runtime values, for
example integers or other objects. In the optimizer however, the
fields of the VirtualObject
store Value
instances, either Constant
instances or Operation
instances.
When the optimizer sees a load
operation, it also removes it, and replaces
the load
with the Operation
(or Constant
) that is stored in the
VirtualObject
at that point:
def optimize_alloc_removal(bb): opt_bb = Block() for op in bb: if op.name == "alloc": op.info = VirtualObject() continue if op.name == "load": info = op.arg(0).info field = get_num(op) op.make_equal_to(info.load(field)) continue if op.name == "store": info = op.arg(0).info field = get_num(op) info.store(field, op.arg(2)) continue opt_bb.append(op) return opt_bb
This is the first version of the optimization. It doesn't handle all kinds of difficult cases, and we'll have to do something about its optimism. But, already in this minimalistic form, we can write a slightly more complicated test with two allocations, one object pointing to the other. It works correctly too, both allocations are removed:
def test_remove_two_allocations(): bb = Block() var0 = bb.getarg(0) obj0 = bb.alloc() sto1 = bb.store(obj0, 0, var0) obj1 = bb.alloc() sto2 = bb.store(obj1, 0, obj0) var1 = bb.load(obj1, 0) var2 = bb.load(var1, 0) bb.print(var2) # the virtual objects look like this: # obj0 # ┌──────┐ # │ 0: ╷ │ # └────┼─┘ # │ # ▼ # obj1 # ┌─────────┐ # │ 0: var0 │ # └─────────┘ # therefore # var1 is the same as obj0 # var2 is the same as var0 opt_bb = optimize_alloc_removal(bb) assert bb_to_str(opt_bb, "optvar") == """\ optvar0 = getarg(0) optvar1 = print(optvar0)"""
Version 2: Re-Materializing Allocations
To make it easier to talk about how the optimizer operates, let's introduce
some terminology. As already seen by the choice
of the class name VirtualObject
, we will call an object virtual if the
optimizer has optimized away the alloc
operation that creates the object.
Other objects are equivalently not virtual, for example those that have
existed before we enter the current code block.
The first problem that we need to fix is the assumption that every allocation can be removed. So far we only looked at small programs where every allocation could be removed, or equivalently, where every object is virtual. A program that creates virtual objects, stores into and loads from them, and then forgets the objects. In this simple case removing the allocations is fine. As we saw in the previous section, it's also fine to have a virtual object reference another virtual, both allocations can be removed.
What are the cases were we can't remove an allocation? The first version of the optimizer simply assumed that every allocation can be removed. This can't work. We will replace this assumption with the following simple heuristic:
If a reference to a virtual object a
is stored into an object b
that is not virtual, then a
will also stop being virtual. If an object a
that was virtual stops being virtual, we say that it escapes. ¹
The simplest test case for this happening looks like this:
def test_materialize(): bb = Block() var0 = bb.getarg(0) obj = bb.alloc() sto = bb.store(var0, 0, obj) opt_bb = optimize_alloc_removal(bb) # obj is virtual, without any fields # ┌───────┐ # │ empty │ # └───────┘ # then we store a reference to obj into # field 0 of var0. Since var0 is not virtual, # obj escapes, so we have to put it back # into the optimized basic block assert bb_to_str(opt_bb, "optvar") == """\ optvar0 = getarg(0) optvar1 = alloc() optvar2 = store(optvar0, 0, optvar1)""" # so far, fails like this: # the line: # info.store(field, op.arg(2)) # produces an AttributeError because info # is None
If the optimizer reaches a point where a virtual object escapes (like the
store
operation in the test), the optimizer has already removed the alloc
operation that created the virtual object. If the object escapes, we don't want
to go back in the operations list and re-insert the alloc
operation, that
sounds potentially very complicated. Instead, we re-insert the alloc
operation that will recreate the virtual object at the point of escape using a
helper function materialize
.
def materialize(opt_bb, value: Operation) -> None: assert not isinstance(value, Constant) assert isinstance(value, Operation) info = value.info assert isinstance(info, VirtualObject) assert value.name == "alloc" # put the alloc operation back into the trace opt_bb.append(value)
I've added a number of fairly strong assertions to materialize
to encode our
current assumptions about the situations in which it expects to be called. We
will remove some of them later as we generalize the code.
Now that we have materialize
we need to change optimize_alloc_removal
to
recognize the case of storing a virtual object into a non-virtual one. We can
recognize Operation
instances that produced a virtual object by looking at
their .info
field. If it is None
, the object is not virtual, otherwise
it is. If we store something into a virtual object, we leave the code as above.
If we store a virtual object into an object that is not virtual, we will first
materialize the virtual object, and then emit the store.
def optimize_alloc_removal(bb): opt_bb = Block() for op in bb: if op.name == "alloc": op.info = VirtualObject() continue if op.name == "load": info = op.arg(0).info field = get_num(op) op.make_equal_to(info.load(field)) continue if op.name == "store": info = op.arg(0).info if info: # virtual field = get_num(op) info.store(field, op.arg(2)) continue else: # not virtual # first materialize the # right hand side materialize(opt_bb, op.arg(2)) # then emit the store via # the general path below opt_bb.append(op) return opt_bb
This is the general idea, and it is enough to pass test_materialize
. But of
course there are still a number of further problems that we now need to solve.
Version 3: Don't Materialize Twice
The first problem is the fact that after we materialize a virtual object, it is
no longer virtual. So if it escapes a second time, it should not be
materialized a second time. A test for that case could simply repeat the
store
operation:
def test_dont_materialize_twice(): # obj is again an empty virtual object, # and we store it into var0 *twice*. # this should only materialize it once bb = Block() var0 = bb.getarg(0) obj = bb.alloc() sto0 = bb.store(var0, 0, obj) sto1 = bb.store(var0, 0, obj) opt_bb = optimize_alloc_removal(bb) assert bb_to_str(opt_bb, "optvar") == """\ optvar0 = getarg(0) optvar1 = alloc() optvar2 = store(optvar0, 0, optvar1) optvar3 = store(optvar0, 0, optvar1)""" # fails so far: the operations that we get # at the moment are: # optvar0 = getarg(0) # optvar1 = alloc() # optvar2 = store(optvar0, 0, optvar1) # optvar3 = alloc() # optvar4 = store(optvar0, 0, optvar3) # ie the object is materialized twice, # which is incorrect
We solve the problem by setting the .info
field of an object that we
materialize to None
to mark it as no longer being virtual.
def materialize(opt_bb, value: Operation) -> None: assert not isinstance(value, Constant) assert isinstance(value, Operation) info = value.info if info is None: return # already materialized assert value.name == "alloc" # put the alloc operation back into the trace opt_bb.append(value) # but only once value.info = None # optimize_alloc_removal unchanged
This fixes the problem, only one alloc
is created. This fix also allows
another test case to pass, one where we store a non-virtual into another
non-virtual, code which we cannot optimize at all:
def test_materialize_non_virtuals(): # in this example we store a non-virtual var1 # into another non-virtual var0 # this should just lead to no optimization at # all bb = Block() var0 = bb.getarg(0) var1 = bb.getarg(1) sto = bb.store(var0, 0, var1) opt_bb = optimize_alloc_removal(bb) assert bb_to_str(opt_bb, "optvar") == """\ optvar0 = getarg(0) optvar1 = getarg(1) optvar2 = store(optvar0, 0, optvar1)"""
Version 4: Materialization of Constants
Another straightforward extension is to support materializing constants. A constant is never virtual, so materializing it should do nothing.
def test_materialization_constants(): # in this example we store the constant 17 # into the non-virtual var0 # again, this will not be optimized bb = Block() var0 = bb.getarg(0) sto = bb.store(var0, 0, 17) opt_bb = optimize_alloc_removal(bb) # the previous line fails so far, triggering # the assert: # assert not isinstance(value, Constant) # in materialize assert bb_to_str(opt_bb, "optvar") == """\ optvar0 = getarg(0) optvar1 = store(optvar0, 0, 17)"""
To implement that case, we check for value
being a constant and return
early:
def materialize(opt_bb, value: Operation) -> None: if isinstance(value, Constant): return assert isinstance(value, Operation) info = value.info if info is None: return # already materialized assert value.name == "alloc" # put the alloc operation back into the trace opt_bb.append(value) # but only once value.info = None # optimize_alloc_removal unchanged
Version 5: Materializing Fields
Now we need to solve a more difficult problem. So far, the virtual objects that we have materialized have all been empty, meaning they didn't have any fields written to at the point of materialization. Let's write a test for this:
def test_materialize_fields(): bb = Block() var0 = bb.getarg(0) var1 = bb.getarg(1) obj = bb.alloc() contents0 = bb.store(obj, 0, 8) contents1 = bb.store(obj, 1, var1) sto = bb.store(var0, 0, obj) # the virtual obj looks like this # obj # ┌──────┬──────────┐ # │ 0: 8 │ 1: var1 │ # └──────┴──────────┘ # then it needs to be materialized # this is the first example where a virtual # object that we want to materialize has any # content and is not just an empty object opt_bb = optimize_alloc_removal(bb) assert bb_to_str(opt_bb, "optvar") == """\ optvar0 = getarg(0) optvar1 = getarg(1) optvar2 = alloc() optvar3 = store(optvar2, 0, 8) optvar4 = store(optvar2, 1, optvar1) optvar5 = store(optvar0, 0, optvar2)""" # fails so far! the operations we get # at the moment are: # optvar0 = getarg(0) # optvar1 = getarg(1) # optvar2 = alloc() # optvar3 = store(optvar0, 0, optvar2) # which is wrong, because the store operations # into optvar1 got lost
To fix this problem, we need to re-create a store
operation for every
element of the .contents
dictionary of the virtual object we are
materializing. ²
def materialize(opt_bb, value: Operation) -> None: if isinstance(value, Constant): return assert isinstance(value, Operation) info = value.info if info is None: return # already materialized assert value.name == "alloc" # put the alloc operation back into the trace opt_bb.append(value) # put the content back for idx, val in info.contents.items(): # re-create store operation opt_bb.store(value, idx, val) # only materialize once value.info = None # optimize_alloc_removal unchanged
This is enough to pass the test.
Version 6: Recursive Materialization
In the above example, the fields of the virtual objects contained only constants or non-virtual objects. However, we could have a situation where a whole tree of virtual objects is built, and then the root of the tree escapes. This makes it necessary to escape the whole tree. Let's write a test for a small tree of two virtual objects:
def test_materialize_chained_objects(): bb = Block() var0 = bb.getarg(0) obj0 = bb.alloc() obj1 = bb.alloc() contents = bb.store(obj0, 0, obj1) const = bb.store(obj1, 0, 1337) sto = bb.store(var0, 0, obj0) # obj0 # ┌──────┐ # │ 0: ╷ │ # └────┼─┘ # │ # ▼ # obj1 # ┌─────────┐ # │ 0: 1337 │ # └─────────┘ # now obj0 escapes opt_bb = optimize_alloc_removal(bb) assert bb_to_str(opt_bb, "optvar") == """\ optvar0 = getarg(0) optvar1 = alloc() optvar2 = alloc() optvar3 = store(optvar2, 0, 1337) optvar4 = store(optvar1, 0, optvar2) optvar5 = store(optvar0, 0, optvar1)""" # fails in an annoying way! the resulting # basic block is not in proper SSA form # so printing it fails. The optimized # block would look like this: # optvar0 = getarg(0) # optvar1 = alloc() # optvar3 = store(optvar1, 0, optvar2) # optvar4 = store(optvar0, 0, optvar1) # where optvar2 is an ``alloc`` Operation # that is not itself in the output block
To fix it, materialize
needs to call itself recursively for all the field
values of the virtual object:
def materialize(opt_bb, value: Operation) -> None: if isinstance(value, Constant): return assert isinstance(value, Operation) info = value.info if info is None: return # already materialized assert value.name == "alloc" # put the alloc operation back into the trace opt_bb.append(value) # put the content back for idx, val in sorted(info.contents.items()): # materialize recursively materialize(opt_bb, val) opt_bb.store(value, idx, val) # only materialize once value.info = None # optimize_alloc_removal unchanged
Getting there, the materialization logic is almost done. We need to fix a subtle remaining problem though.
Version 7: Dealing with Object Cycles
The bug we need to fix in this section is a bit tricky, and does not immediately occur in a lot of programs. In fact, in PyPy a variant of it was hiding out in our optimizer until we found it much later (despite us being aware of the general problem and correctly dealing with it in other cases).
The problem is this: a virtual object can (directly or indirectly) point to
itself, and we must carefully deal with that case to avoid infinite recursion in
materialize
. Here's the simplest test:
def test_object_graph_cycles(): bb = Block() var0 = bb.getarg(0) var1 = bb.alloc() var2 = bb.store(var1, 0, var1) var3 = bb.store(var0, 1, var1) # ┌────────┐ # ▼ │ # obj0 │ # ┌──────┐ │ # │ 0: ╷ │ │ # └────┼─┘ │ # │ │ # └─────┘ # obj0 points to itself, and then it is # escaped opt_bb = optimize_alloc_removal(bb) # the previous line fails with an # InfiniteRecursionError # materialize calls itself, infinitely # what we want is instead this output: assert bb_to_str(opt_bb, "optvar") == """\ optvar0 = getarg(0) optvar1 = alloc() optvar2 = store(optvar1, 0, optvar1) optvar3 = store(optvar0, 1, optvar1)"""
The fix is not a big change, but a little bit subtle nevertheless.
We have to change the
order in which things are done in materialize
. Right after emitting the
alloc
, we set the .info
to None
, to mark the object as not virtual.
Only afterwards do we re-create the stores and call materialize
recursively.
If a recursive call reaches the same object, it's already marked as non-virtual,
so materialize
won't recurse further:
def materialize(opt_bb, value: Operation) -> None: if isinstance(value, Constant): return assert isinstance(value, Operation) info = value.info if info is None: return # already materialized assert value.name == "alloc" # put the alloc operation back into the trace opt_bb.append(value) # only materialize once value.info = None # put the content back for idx, val in sorted(info.contents.items()): # materialize recursively materialize(opt_bb, val) opt_bb.store(value, idx, val)
Version 8: Loading from non-virtual objects
Now materialize is done. We need to go back to optimize_alloc_removal
and
improve it further. The last time we changed it, we added a case analysis to the
code dealing with store
, distinguishing between storing to a virtual and to
a non-virtual object. We need to add an equivalent distinction to the load
case, because right now loading from a non-virtual crashes.
def test_load_non_virtual(): bb = Block() var0 = bb.getarg(0) var1 = bb.load(var0, 0) bb.print(var1) # the next line fails in the line # op.make_equal_to(info.load(field)) # because info is None opt_bb = optimize_alloc_removal(bb) assert bb_to_str(opt_bb, "optvar") == """\ optvar0 = getarg(0) optvar1 = load(optvar0, 0) optvar2 = print(optvar1)"""
To fix it, we split the load
code into two cases, leaving the virtual path
as before, and letting the load
from a non-virtual fall through to the
general code at the end of the function.
def optimize_alloc_removal(bb): opt_bb = Block() for op in bb: if op.name == "alloc": op.info = VirtualObject() continue if op.name == "load": info = op.arg(0).info if info: # virtual field = get_num(op) op.make_equal_to(info.load(field)) continue # otherwise not virtual, use the # general path below if op.name == "store": info = op.arg(0).info if info: # virtual field = get_num(op) info.store(field, op.arg(2)) continue else: # not virtual # first materialize the # right hand side materialize(opt_bb, op.arg(2)) # then emit the store via # the general path below opt_bb.append(op) return opt_bb
Version 9 (Final): Materialize on Other Operations
We're almost at the end now. There's one final generalization left to do. We
started with the heuristic that storing a virtual into a non-virtual would
escape it. This should be generalized. Every time we pass a virtual into any
operation where it is not the first argument of a load
and a store
should also escape it (imagine passing the virtual to some function call).
Let's test this as usual with our print
operation:
def test_materialize_on_other_ops(): # materialize not just on store bb = Block() var0 = bb.getarg(0) var1 = bb.alloc() var2 = bb.print(var1) opt_bb = optimize_alloc_removal(bb) assert bb_to_str(opt_bb, "optvar") == """\ optvar0 = getarg(0) optvar1 = alloc() optvar2 = print(optvar1)""" # again, the resulting basic block is not in # valid SSA form
To fix this, we will take the call to materialize
out of the store
code
path and instead put it into the generic code path the end of the while
loop:
# materialize is unchanged def materialize(opt_bb, value: Value) -> None: if isinstance(value, Constant): return assert isinstance(value, Operation) info = value.info if not info: # Already materialized return assert value.name == "alloc" opt_bb.append(value) value.info = None for idx, val in sorted(info.contents.items()): materialize(opt_bb, val) opt_bb.store(value, idx, val) def optimize_alloc_removal(bb): opt_bb = Block() for op in bb: if op.name == "alloc": op.info = VirtualObject() continue if op.name == "load": info = op.arg(0).info if info: # virtual field = get_num(op) op.make_equal_to(info.load(field)) continue if op.name == "store": info = op.arg(0).info if info: # virtual field = get_num(op) info.store(field, op.arg(2)) continue # materialize all the arguments of # operations that are put into the # output basic block for arg in op.args: materialize(opt_bb, arg.find()) opt_bb.append(op) return opt_bb
That's it, we're done. It's not a lot of code, but actually quite a powerful optimization. In addition to removing allocations for objects that are only used briefly and in predictable ways, it also has another effect. If an object is allocated, used in a number of operations and then escapes further down in the block, the operations in between can often be optimized away. This is demonstrated by the next test (which already passes):
def test_sink_allocations(): bb = Block() var0 = bb.getarg(0) var1 = bb.alloc() var2 = bb.store(var1, 0, 123) var3 = bb.store(var1, 1, 456) var4 = bb.load(var1, 0) var5 = bb.load(var1, 1) var6 = bb.add(var4, var5) var7 = bb.store(var1, 0, var6) var8 = bb.store(var0, 1, var1) opt_bb = optimize_alloc_removal(bb) assert bb_to_str(opt_bb, "optvar") == """\ optvar0 = getarg(0) optvar1 = add(123, 456) optvar2 = alloc() optvar3 = store(optvar2, 0, optvar1) optvar4 = store(optvar2, 1, 456) optvar5 = store(optvar0, 1, optvar2)"""
Note that the addition is not optimized away, because the code from this blog post does not contain constant folding and the other optimizations from the last one. Combining them would not be too hard though.
Conclusion
That's it! The core idea of PyPy's allocation removal optimization in one or two screens of code. The real implementation has a number of refinements, but the core ideas are all here.
I'm not going to show any benchmark numbers or anything like that here, if you are interested in numbers you could look at the evaluation Section 6. "Implementation and Evaluation" of the paper that describes the work.
There's a complementary optimization that improves load
and store
operations for objects that are not virtual. I'll probably not write that
down as another post, but Max Bernstein and I developed that together on a
PyPy Twitch channel channel a few weeks ago, here's the recording:
Footnotes
¹ This is how PyPy uses the terminology, not really used consistently by other projects. The term "escape" is fairly standard throughout the escape analysis literature. The term "virtual" was used originally in Armin Rigo's Psyco but is e.g. also used by the paper Partial Escape Analysis and Scalar Replacement for Java.
² The order in which we put the store operations back is relying on dictionary iteration order, which is insertion order. That's not a bad ordering, we could also be explicit and sort the fields in some order (ideally the order in which the object lays them out in memory).
Düsseldorf HPy/PyPy/GraalPy sprint September 19-23rd 2022
The programming language group of the Computer Science department of Heinrich-Heine Universität Düsseldorf is happy to invite everybody to another sprint in Düsseldorf, from the 19th to the 23rd of September 2022. This is a fully public sprint, everyone and particularly newcomers are welcome to join us! The goal is to bring together people from the HPy, PyPy, GraalPy and CPython communities.
Topics and goals
work on HPy APIs, discussions around next steps for the project
continuing new and ongoing ports to HPy, including Cython, NumPy, Pillow, Matplotlib
3.10 support on PyPy and GraalPy
preparing the next PyPy release
discussions around ways to improve collaboration between the different Python implementations
What is a sprint?
The experience of the PyPy project has shown the benefits of regular sprints. They are focussed one week physical meetings where people pair-program on new features and discuss future plans. Coming to one is a great way to get started with a project!
Location
The sprint will take place in a seminar room of the computer science department. It is in the building 25.12, room 02.50 (second floor) of the university campus. For travel instructions see
We ask participants to wear masks during the indoor working hours.

Wiegels, CC BY 3.0, via Wikimedia Commons
Exact times
Work days: starting September 19th (~morning), ending September 23rd (~afternoon). We will do a to-be-planned social activity on Wednesday afternoon.
Registration
Please register by editing this file or by opening a pull request:
https://foss.heptapod.net/pypy/extradoc/-/blob/branch/extradoc/sprintinfo/ddorf2022/people.txt
or by sending a quick mail to the pypy-dev mailing list:
M1 support for PyPy
The PyPy team is happy to announce that we can now target the macOS ARM64
platform. Much of the work was executed by Maciej Fijałkowski (fijal) and
funded via a generous contribution to our OpenCollective. The work is based
on our existing support for aarch64 (arm64 on linux) with some twists
to support the differences between the CPUs and the operating system. There
are nightly builds for pypy3.8 and pypy3.9 (look for macos_arm64
), and
the architecture will be part of our next release.
Please try it out and let us know how it is useful for you or how we could improve.
We still need help improving our macOS support. We have an open issue to help our packaging story. Help is welcome.
The PyPy team.
Implementing a Toy Optimizer
In this blog post I want to show the complete code (in Python3) of how a very simple optimizer for sequences of operations can work. These algorithms could be part of a (really simple) compiler, or a JIT. The architecture of the code in this blog post is very similar to that of the trace optimizer of the PyPy JIT: After a trace is produced, is is optimized before being sent to the machine code backend that produces binary instructions for the CPU architecture that PyPy is running on.
To get started, the first thing we need to do is define how our operations are stored. The format that a compiler uses to store the program while it is being optimized is usually called its intermediate representation (IR). Many production compilers use IRs that are in the Static Single-Assignment Form (SSA), and we will also use that. SSA form has the property that every variable is assigned to exactly once, and every variable is defined before it is used. This simplifies many things.
Let's make this concrete. If our input program is a complex expressions, such
as a * (b + 17) + (b + 17)
the intermediate representation of that (or at
least its text representation) would maybe be something like:
var1 = add(b, 17) var2 = mul(a, var1) var3 = add(b, 17) var4 = add(var2, var3)
This sequence of instructions is inefficient. The operation add(b, 17)
is
computed twice and we can save time by removing the second one and only
computing it once. In this post I want to show an optimizer that can do this
(and some related) optimizations.
Looking at the IR we notice that the input expression has been linearized into a sequence of operations, and all the intermedia results have been given unique variable names. The value that every variable is assigned is computed by the right hand side, which is some operation consisting of an operand and an arbitrary number of arguments. The arguments of an operation are either themselves variables or constants.
I will not at all talk about the process of translating the input program into the IR. Instead, I will assume we have some component that does this translation already. The tests in this blog post will construct small snippets of IR by hand. I also won't talk about what happens after the optimization (usually the optimized IR is translated into machine code).
Implementing the Intermediate Representation
Let's start modelling the intermediate representation with Python classes. First we define a base class of all values that can be used as arguments in operations, and let's also add a class that represents constants:
import pytest from typing import Optional, Any class Value: pass class Constant(Value): def __init__(self, value: Any): self.value = value def __repr__(self): return f"Constant({self.value})"
One consequence of the fact that every variable is assigned to only once is that variables are in a one-to-one correspondence with the right-hand-side of their unique assignments. That means that we don't need a class that represents variables at all. Instead, it's sufficient to have a class that represents an operation (the right-hand side), and that by definition is the same as the variable (left-hand side) that it defines:
class Operation(Value): def __init__(self, name: str, args: list[Value]): self.name = name self.args = args def __repr__(self): return f"Operation({self.name}, {self.args})" def arg(self, index: int): return self.args[index]
Now we can instantiate these two classes to represent the example sequence of operations above:
def test_construct_example(): # first we need something to represent # "a" and "b". In our limited view, we don't # know where they come from, so we will define # them with a pseudo-operation called "getarg" # which takes a number n as an argument and # returns the n-th input argument. The proper # SSA way to do this would be phi-nodes. a = Operation("getarg", [Constant(0)]) b = Operation("getarg", [Constant(1)]) # var1 = add(b, 17) var1 = Operation("add", [b, Constant(17)]) # var2 = mul(a, var1) var2 = Operation("mul", [a, var1]) # var3 = add(b, 17) var3 = Operation("add", [b, Constant(17)]) # var4 = add(var2, var3) var4 = Operation("add", [var2, var3]) sequence = [a, b, var1, var2, var3, var4] # nothing to test really, it shouldn't crash
Usually, complicated programs are represented as a control flow graph in a compiler, which represents all the possible paths that control can take while executing the program. Every node in the control flow graph is a basic block. A basic block is a linear sequence of operations with no control flow inside of it.
When optimizing a program, a compiler usually looks at the whole control flow graph of a function. However, that is still too complicated! So let's simplify further and look at only at optimizations we can do when looking at a single basic block and its sequence of instructions (they are called local optimizations).
Let's define a class representing basic blocks and let's also add some
convenience functions for constructing sequences of operations, because the
code in test_construct_example
is a bit annoying.
class Block(list): def opbuilder(opname): def wraparg(arg): if not isinstance(arg, Value): arg = Constant(arg) return arg def build(self, *args): # construct an Operation, wrap the # arguments in Constants if necessary op = Operation(opname, [wraparg(arg) for arg in args]) # add it to self, the basic block self.append(op) return op return build # a bunch of operations we support add = opbuilder("add") mul = opbuilder("mul") getarg = opbuilder("getarg") dummy = opbuilder("dummy") lshift = opbuilder("lshift") def test_convencience_block_construction(): bb = Block() # a again with getarg, the following line # defines the Operation instance and # immediately adds it to the basic block bb a = bb.getarg(0) assert len(bb) == 1 assert bb[0].name == "getarg" # it's a Constant assert bb[0].args[0].value == 0 # b with getarg b = bb.getarg(1) # var1 = add(b, 17) var1 = bb.add(b, 17) # var2 = mul(a, var1) var2 = bb.mul(a, var1) # var3 = add(b, 17) var3 = bb.add(b, 17) # var4 = add(var2, var3) var4 = bb.add(var2, var3) assert len(bb) == 6
That's a good bit of infrastructure to make the tests easy to write. One
thing we are lacking though is a way to print the basic blocks into a nicely
readable textual representation. Because in the current form, the repr
of a
Block is very annoying, the output of pretty-printing bb
in the test above
looks like this:
[Operation('getarg', [Constant(0)]), Operation('getarg', [Constant(1)]), Operation('add', [Operation('getarg', [Constant(1)]), Constant(17)]), Operation('mul', [Operation('getarg', [Constant(0)]), Operation('add', [Operation('getarg', [Constant(1)]), Constant(17)])]), Operation('add', [Operation('getarg', [Constant(1)]), Constant(17)]), Operation('add', [Operation('mul', [Operation('getarg', [Constant(0)]), Operation('add', [Operation('getarg', [Constant(1)]), Constant(17)])]), Operation('add', [Operation('getarg', [Constant(1)]), Constant(17)])])]
It's impossible to see what is going on here, because the Operations
in the
basic block appear several times, once as elements of the list but then also as
arguments to operations further down in the list. So we need some code that
turns things back into a readable textual representation, so we have a chance
to debug.
def bb_to_str(bb: Block, varprefix: str = "var"): # the implementation is not too important, # look at the test below to see what the # result looks like def arg_to_str(arg: Value): if isinstance(arg, Constant): return str(arg.value) else: # the key must exist, otherwise it's # not a valid SSA basic block: # the variable must be defined before # its first use return varnames[arg] varnames = {} res = [] for index, op in enumerate(bb): # give the operation a name used while # printing: var = f"{varprefix}{index}" varnames[op] = var arguments = ", ".join( arg_to_str(op.arg(i)) for i in range(len(op.args)) ) strop = f"{var} = {op.name}({arguments})" res.append(strop) return "\n".join(res) def test_basicblock_to_str(): bb = Block() var0 = bb.getarg(0) var1 = bb.add(5, 4) var2 = bb.add(var1, var0) assert bb_to_str(bb) == """\ var0 = getarg(0) var1 = add(5, 4) var2 = add(var1, var0)""" # with a different prefix for the invented # variable names: assert bb_to_str(bb, "x") == """\ x0 = getarg(0) x1 = add(5, 4) x2 = add(x1, x0)""" # and our running example: bb = Block() a = bb.getarg(0) b = bb.getarg(1) var1 = bb.add(b, 17) var2 = bb.mul(a, var1) var3 = bb.add(b, 17) var4 = bb.add(var2, var3) assert bb_to_str(bb, "v") == """\ v0 = getarg(0) v1 = getarg(1) v2 = add(v1, 17) v3 = mul(v0, v2) v4 = add(v1, 17) v5 = add(v3, v4)""" # Note the re-numbering of the variables! We # don't attach names to Operations at all, so # the printing will just number them in # sequence, can sometimes be a source of # confusion.
This is much better. Now we're done with the basic infrastructure, we can define sequences of operations and print them in a readable way. Next we need a central data structure that is used when actually optimizing basic blocks.
Storing Equivalences between Operations Using a Union-Find Data Structure
When optimizing a sequence of operations, we want to make it less costly to
execute. For that we typically want to remove operations (and sometimes
replace operations with less expensive ones). We can remove operations if
they do redundant computation, like case of the duplicate add(v1, 17)
in
the example. So what we want to do is to turn the running input sequence:
v0 = getarg(0) v1 = getarg(1) v2 = add(v1, 17) v3 = mul(v0, v2) v4 = add(v1, 17) v5 = add(v3, v4)
Into the following optimized output sequence:
optvar0 = getarg(0) optvar1 = getarg(1) optvar2 = add(optvar1, 17) optvar3 = mul(optvar0, optvar2) optvar4 = add(optvar3, optvar2)
We left out the second add
(which defines v4
), and then replaced the
usage of v4
with v2
in the final operation that defines v5
.
What we effectively did was discover that v2
and v4
are equivalent and then
replaced v4
with v2
. In general, we might discover more such equivalences,
and we need a data structure to store them. A good data structure to store
these equivalences is Union Find (also called Disjoint-set data structure),
which stores a collection of disjoint sets. Disjoint means, that no operation
can appear in more than one set. The sets in our concrete case are the sets of
operations that compute the same result.
When we start out, every operation is in its own singleton set, with no other
member. As we discover more equivalences, we will unify sets into larger sets
of operations that all compute the same result. So one operation the data
structure supports is union
, to unify two sets, we'll call that
make_equal_to
in the code below.
The other operation the data structure supports is find
, which takes an
operation and returns a "representative" of the set of all equivalent
operations. Two operations are in the same set, if the representative that
find returns for them is the same.
The exact details of how the data structure works are only sort of important
(even though it's very cool, I promise!). It's OK to skip over the
implementation. We will add the data structure right into our Value
,
Constant
and Operation
classes:
class Value: def find(self): raise NotImplementedError("abstract") def _set_forwarded(self, value): raise NotImplementedError("abstract") class Operation(Value): def __init__(self, name: str, args: list[Value]): self.name = name self.args = args self.forwarded = None def __repr__(self): return ( f"Operation({self.name}," f"{self.args}, {self.forwarded})" ) def find(self) -> Value: # returns the "representative" value of # self, in the union-find sense op = self while isinstance(op, Operation): # could do path compression here too # but not essential next = op.forwarded if next is None: return op op = next return op def arg(self, index): # change to above: return the # representative of argument 'index' return self.args[index].find() def make_equal_to(self, value: Value): # this is "union" in the union-find sense, # but the direction is important! The # representative of the union of Operations # must be either a Constant or an operation # that we know for sure is not optimized # away. self.find()._set_forwarded(value) def _set_forwarded(self, value: Value): self.forwarded = value class Constant(Value): def __init__(self, value: Any): self.value = value def __repr__(self): return f"Constant({self.value})" def find(self): return self def _set_forwarded(self, value: Value): # if we found out that an Operation is # equal to a constant, it's a compiler bug # to find out that it's equal to another # constant assert isinstance(value, Constant) and \ value.value == self.value def test_union_find(): # construct three operation, and unify them # step by step bb = Block() a1 = bb.dummy(1) a2 = bb.dummy(2) a3 = bb.dummy(3) # at the beginning, every op is its own # representative, that means every # operation is in a singleton set # {a1} {a2} {a3} assert a1.find() is a1 assert a2.find() is a2 assert a3.find() is a3 # now we unify a2 and a1, then the sets are # {a1, a2} {a3} a2.make_equal_to(a1) # they both return a1 as the representative assert a1.find() is a1 assert a2.find() is a1 # a3 is still different assert a3.find() is a3 # now they are all in the same set {a1, a2, a3} a3.make_equal_to(a2) assert a1.find() is a1 assert a2.find() is a1 assert a3.find() is a1 # now they are still all the same, and we # also learned that they are the same as the # constant 6 # the single remaining set then is # {6, a1, a2, a3} c = Constant(6) a2.make_equal_to(c) assert a1.find() is c assert a2.find() is c assert a3.find() is c # union with the same constant again is fine a2.make_equal_to(c)
Constant Folding
Now comes the first actual optimization, a simple constant folding pass. It will remove operations where all the arguments are constants and replace them with the constant result.
Every pass has the same structure: we go over all operations in the basic
block in order and decide for each operation whether it can be removed. For the
constant folding pass, we can remove all the operations with constant
arguments (but we'll implement only the add
case here).
I will show a buggy version of the constant folding pass first. It has a problem that is related to why we need the union-find data structure. We will fix it a bit further down.
def constfold_buggy(bb: Block) -> Block: opt_bb = Block() for op in bb: # basic idea: go over the list and do # constant folding of add where possible if op.name == "add": arg0 = op.args[0] arg1 = op.args[1] if isinstance(arg0, Constant) and \ isinstance(arg1, Constant): # can constant-fold! that means we # learned a new equality, namely # that op is equal to a specific # constant value = arg0.value + arg1.value op.make_equal_to(Constant(value)) # don't need to have the operation # in the optimized basic block continue # otherwise the operation is not # constant-foldable and we put into the # output list opt_bb.append(op) return opt_bb def test_constfold_simple(): bb = Block() var0 = bb.getarg(0) var1 = bb.add(5, 4) var2 = bb.add(var1, var0) opt_bb = constfold_buggy(bb) assert bb_to_str(opt_bb, "optvar") == """\ optvar0 = getarg(0) optvar1 = add(9, optvar0)""" @pytest.mark.xfail def test_constfold_buggy_limitation(): # this test fails! it shows the problem with # the above simple constfold_buggy pass bb = Block() var0 = bb.getarg(0) # this is folded var1 = bb.add(5, 4) # we want this folded too, but it doesn't work var2 = bb.add(var1, 10) var3 = bb.add(var2, var0) opt_bb = constfold_buggy(bb) assert bb_to_str(opt_bb, "optvar") == """\ optvar0 = getarg(0) optvar1 = add(19, optvar0)"""
Why does the test fail? The opt_bb
printed output looks like this:
optvar0 = getarg(0) optvar1 = add(9, 10) optvar2 = add(optvar1, optvar0)
The problem is that when we optimize the second addition in constfold_buggy,
the argument of that operation is an Operation not a Constant
, so
constant-folding is not applied to the second add. However, we have already
learned that the argument var1
to the operation var2
is equal to
Constant(9)
. This information is stored in the union-find data structure.
So what we are missing are suitable find calls in the constant folding pass, to
make use of the previously learned equalities.
Here's the fixed version:
def constfold(bb: Block) -> Block: opt_bb = Block() for op in bb: # basic idea: go over the list and do # constant folding of add where possible if op.name == "add": # >>> changed arg0 = op.arg(0) # uses .find() arg1 = op.arg(1) # uses .find() # <<< end changes if isinstance(arg0, Constant) and \ isinstance(arg1, Constant): # can constant-fold! that means we # learned a new equality, namely # that op is equal to a specific # constant value = arg0.value + arg1.value op.make_equal_to(Constant(value)) # don't need to have the operation # in the optimized basic block continue # otherwise the operation is not # constant-foldable and we put into the # output list opt_bb.append(op) return opt_bb def test_constfold_two_ops(): # now it works! bb = Block() var0 = bb.getarg(0) var1 = bb.add(5, 4) var2 = bb.add(var1, 10) var3 = bb.add(var2, var0) opt_bb = constfold(bb) assert bb_to_str(opt_bb, "optvar") == """\ optvar0 = getarg(0) optvar1 = add(19, optvar0)"""
Common Subexpression Elimination
The constfold
pass only discovers equalities between Operations
and
Constants
. Let's do a second pass that also discovers equalities between
Operations
and other Operations
.
A simple optimization that does that has this property common subexpression elimination (CSE), which will finally optimize away the problem in the introductory example code that we had above.
def cse(bb: Block) -> Block: # structure is the same, loop over the input, # add some but not all operations to the # output opt_bb = Block() for op in bb: # only do CSE for add here, but it # generalizes if op.name == "add": arg0 = op.arg(0) arg1 = op.arg(1) # Check whether we have emitted the # same operation already prev_op = find_prev_add_op( arg0, arg1, opt_bb) if prev_op is not None: # if yes, we can optimize op away # and replace it with the earlier # result, which is an Operation # that was already emitted to # opt_bb op.make_equal_to(prev_op) continue opt_bb.append(op) return opt_bb def eq_value(val0, val1): if isinstance(val0, Constant) and \ isinstance(val1, Constant): # constants compare by their value return val0.value == val1.value # everything else by identity return val0 is val1 def find_prev_add_op(arg0: Value, arg1: Value, opt_bb: Block) -> Optional[Operation]: # Really naive and quadratic implementation. # What we do is walk over the already emitted # operations and see whether we emitted an add # with the current arguments already. A real # implementation might use a hashmap of some # kind, or at least only look at a limited # window of instructions. for opt_op in opt_bb: if opt_op.name != "add": continue # It's important to call arg here, # for the same reason why we # needed it in constfold: we need to # make sure .find() is called if eq_value(arg0, opt_op.arg(0)) and \ eq_value(arg1, opt_op.arg(1)): return opt_op return None def test_cse(): bb = Block() a = bb.getarg(0) b = bb.getarg(1) var1 = bb.add(b, 17) var2 = bb.mul(a, var1) var3 = bb.add(b, 17) var4 = bb.add(var2, var3) opt_bb = cse(bb) assert bb_to_str(opt_bb, "optvar") == """\ optvar0 = getarg(0) optvar1 = getarg(1) optvar2 = add(optvar1, 17) optvar3 = mul(optvar0, optvar2) optvar4 = add(optvar3, optvar2)"""
Strength Reduction
Now we have one pass that replaces Operations
with Constants
and one that
replaces Operations
with previously existing Operations
. Let's now do one
final pass that replaces Operations
by newly invented Operations
, a simple
strength reduction. This one will be simple.
def strength_reduce(bb: Block) -> Block: opt_bb = Block() for op in bb: if op.name == "add": arg0 = op.arg(0) arg1 = op.arg(1) if arg0 is arg1: # x + x turns into x << 1 newop = opt_bb.lshift(arg0, 1) op.make_equal_to(newop) continue opt_bb.append(op) return opt_bb def test_strength_reduce(): bb = Block() var0 = bb.getarg(0) var1 = bb.add(var0, var0) opt_bb = strength_reduce(bb) assert bb_to_str(opt_bb, "optvar") == """\ optvar0 = getarg(0) optvar1 = lshift(optvar0, 1)"""
Putting Things Together
Let's combine the passes into one single pass, so that we are going over all the operations only exactly once, instead of having to look at every operation once for all the different passes.
def optimize(bb: Block) -> Block: opt_bb = Block() for op in bb: if op.name == "add": arg0 = op.arg(0) arg1 = op.arg(1) # constant folding if isinstance(arg0, Constant) and \ isinstance(arg1, Constant): value = arg0.value + arg1.value op.make_equal_to(Constant(value)) continue # cse prev_op = find_prev_add_op( arg0, arg1, opt_bb) if prev_op is not None: op.make_equal_to(prev_op) continue # strength reduce: # x + x turns into x << 1 if arg0 is arg1: newop = opt_bb.lshift(arg0, 1) op.make_equal_to(newop) continue # and while we are at it, let's do some # arithmetic simplification: # a + 0 => a if eq_value(arg0, Constant(0)): op.make_equal_to(arg1) continue if eq_value(arg1, Constant(0)): op.make_equal_to(arg0) continue opt_bb.append(op) return opt_bb def test_single_pass(): bb = Block() # constant folding var0 = bb.getarg(0) var1 = bb.add(5, 4) var2 = bb.add(var1, 10) var3 = bb.add(var2, var0) opt_bb = optimize(bb) assert bb_to_str(opt_bb, "optvar") == """\ optvar0 = getarg(0) optvar1 = add(19, optvar0)""" # cse + strength reduction bb = Block() var0 = bb.getarg(0) var1 = bb.getarg(1) var2 = bb.add(var0, var1) var3 = bb.add(var0, var1) # the same as var3 var4 = bb.add(var2, 2) var5 = bb.add(var3, 2) # the same as var4 var6 = bb.add(var4, var5) opt_bb = optimize(bb) assert bb_to_str(opt_bb, "optvar") == """\ optvar0 = getarg(0) optvar1 = getarg(1) optvar2 = add(optvar0, optvar1) optvar3 = add(optvar2, 2) optvar4 = lshift(optvar3, 1)""" # removing + 0 bb = Block() var0 = bb.getarg(0) var1 = bb.add(16, -16) var2 = bb.add(var0, var1) var3 = bb.add(0, var2) var4 = bb.add(var2, var3) opt_bb = optimize(bb) assert bb_to_str(opt_bb, "optvar") == """\ optvar0 = getarg(0) optvar1 = lshift(optvar0, 1)"""
Conclusion
That's it for now. Why is this architecture cool? From a software engineering
point of view, sticking everything into a single function like in optimize
above is obviously not great, and if you wanted to do this for real you would
try to split the cases into different functions that are individually
digestible, or even use a DSL that makes the pattern matching much more
readable. But the advantage of the architecture is that it's quite efficient,
it makes it possible to pack a lot of good optimizations into a single pass
over a basic block.
Of course this works even better if you are in a tracing context, where everything is put into a trace, which is basically one incredibly long basic block. In a JIT context it's also quite important that the optimizer itself runs quickly.
Various other optimizations are possible in this model. There is a follow-up post that show how to implement what is arguably PyPy's most important optimization.
Some Further Pointers
This post is only a short introduction and is taking some shortcuts, I wanted to also give some (non-exhaustive) pointers to more general literature about the touched topics.
The approach to CSE described here is usually can be seen as value numbering, it's normally really implemented with a hashmap though. Here's a paper that describes various styles of implementing that, even beyond a single basic block. The paper also partly takes the perspective of discovering equivalence classes of operations that compute the same result.
A technique that leans even more fully into finding equivalences between operations is using e-graphs and then applying equality saturation (this is significantly more advanced that what I described here though). A cool modern project that applies this technique is egg.
If you squint a bit, you can generally view a constant folding pass as a very simple form of Partial Evaluation: every operation that has constant arguments is constant-folded away, and the remaining ones are "residualized", i.e. put into the output program. This point of view is not super important for the current post, but will become important in the next one.
Acknowledgements: Thanks to Thorsten Ball for getting me to write this and for his enthusiastic feedback. I also got great feedback from Max Bernstein, Matti Picus and Per Vognsen. A conversation with Peng Wu that we had many many years ago and that stuck with me made me keep thinking about various ways to view compiler optimizations.
How is PyPy Tested?
How is PyPy Tested?
In this post I want to give an overview of how the PyPy project does and thinks about testing. PyPy takes testing quite seriously and has done some from the start of the project. Here I want to present the different styles of tests that PyPy has, when we use them and how I think about them.
Background
To make the blog post self-contained, I am going to start with a small overview about PyPy's architecture. If you already know what PyPy is and how it works, you can skip this section.
PyPy means "Python in Python". It is an alternative implementation of the Python language. Usually, when we speak of "Python", we can mean two different things. On the one hand it means "Python as an abstract programming language". On the other hand, the main implementation of that language is also often called "Python". To more clearly distinguish the two, the implementation is often also called "CPython", because it is an interpreter implemented in C code.
Now we can make the statement "PyPy is Python in Python" more precise: PyPy is an interpreter for Python 3.9, implemented in RPython. RPython ("Restricted Python") is a subset of Python 2, which is statically typed (using type inference, not type annotations) and can be compiled to C code. That means we can take our Python 3.9 interpreter, and compile it into a C binary that can run Python 3.9 code. The final binary behaves pretty similarly to CPython.
The main thing that makes PyPy interesting is that during the translation of our interpreter to C, a number of components are automatically inserted into the final binary. One component is a reasonably good garbage collector.
The more exciting component that is inserted into the binary is a just-in-time compiler. The insertion of this component is not fully automatic, instead it is guided by a small number of annotations in the source code of the interpreter. The effect of inserting this JIT compiler into the binary is that the resulting binary can run Python code significantly faster than CPython, in many cases. How this works is not important for the rest of the post, if you want to see an example of concretely doing that to a small interpreter you can look at this video.
PyPy Testing History
A few historical notes on the PyPy project and its relationship to testing: The PyPy project was started in 2004. At the time when the project was started, Extreme Programming and Agile Software Development were up and coming. On the methodology side, PyPy was heavily influenced by these, and started using Test-Driven Development and pair programming right from the start.
Also technologically, PyPy has been influential on testing in the Python world.
Originally, PyPy had used the unittest
testing framework, but pretty soon
the developers got frustrated with it. Holger Krekel, one of the original
developers who started PyPy, started the pytest testing framework soon
afterwards.
Interpreter-Level Tests
So, how are tests for PyPy written, concretely? The tests for the interpreter
are split into two different kinds, which we call "interpreter level tests" and
"application level tests". The former are tests that can be used to test the
objects and functions that are used in the implementation of the Python
interpreter. Since the interpreter is written in Python 2, those tests are also
written in Python 2, using pytest. They tend to be more on the unit test side of
things. They are in files with the pattern test_*.py
.
Here is an example that tests the implementation of integers (very slightly simplified):
class TestW_IntObject: ... def test_hash(self): w_x = W_IntObject(42) w_result = w_x.descr_hash(self.space) assert isinstance(w_result, W_IntObject) assert w_result.intval == 42
This test checks that if you take an object that represents integers in the
Python language (using the class W_IntObject
, a "wrapped integer object")
with the value 42, computing the hash of that object returns another instance of
the same class, also with the value 42.
These tests can be run on top of any Python 2 implementation, either CPython or
PyPy. We can then test and debug the internals of the PyPy interpreter using
familiar tools like indeed pytest and the Python debuggers. They can be run,
because all the involved code like the tests and the class W_IntObject
are
just completely regular Python 2 classes that behave in the regular way when
run on top of a Python interpreter.
In CPython, these tests don't really have an equivalent. They would correspond to tests that are written in C and that can test the logic of all the C functions of CPython that execute certain functionality, accessing the internals of C structs in the process. ¹
Application-Level Tests
There is also a second class of tests for the interpreter. Those are tests that
don't run on the level of the implementation. Instead, they are executed by
the PyPy Python interpreter, thus running on the level of the applications run
by PyPy. Since the interpreter is running Python 3, the tests are also written
in Python 3. They are stored in files with the pattern apptest_*.py
and
look like "regular" Python 3 tests. ²
Here's an example of how you could write a test equivalent to the one above:
This style of test looks more "natural" and is the preferred one in cases where the test does not need to access the internals of the logic or the objects of the interpreter.
Application level tests can be run in two different ways. On the one hand, we can simply run them on CPython 3. This is very useful! Since we want PyPy to behave like CPython, running the tests that we write on CPython is useful to make sure that the tests themselves aren't wrong.
On the other hand, the main way to run these tests is on top of PyPy, itself running on top of a Python 2 implementation. This makes it possible to run the test without first bootstrapping PyPy to C. Since bootstrapping to C is a relatively slow operation (can take up to an hour) it is crucially important to be able to run tests without bootstrapping first. It also again makes it possible to debug crashes in the interpreter using the regular Python 2 debugger. Of course running tests in this way is unfortunately itself not super fast, given that they run on a stack of two different interpreters.
Application-level tests correspond quite closely to CPython's tests suite (which is using the unittest framework). Of course in CPython it is not possible to run the test suite without building the CPython binary using a C compiler. ³
So when do we write application-level tests, and when interpreter-level tests?
Interpreter-level tests are necessary to test internal data structures that
touch data and logic that is not directly exposed to the Python language. If
that is not necessary, we try to write application-level tests. App-level tests
are however by their nature always more on the integration test side of things.
To be able to run the test_hash
function above, many parts of PyPy need to
work correctly, the parser, the bytecode compiler, the bytecode interpreter, the
hash
builtin, calling the __hash__
special method, etc, etc.
This observation is also true for CPython! One could argue that CPython has no unit tests at all, because in order to be able to even run the tests, most of Python needs to be in working order already, so all the tests are really implicitly integration tests.
The CPython Test Suite
We also use the CPython Test suite as a final check to see whether our
interpreter correctly implements all the features of the Python language. In
that sense it acts as some kind of compliance test suite that checks whether we
implement the language correctly. The test suite is not perfect for this.
Since it is written for CPython's purposes during its development, a
lot of the tests check really specific CPython implementation details. Examples
for these are tests that check that __del__
is called immediately after
objects go out of scope (which only happens if you use reference counting as a
garbage collection strategy, PyPy uses a different approach to garbage
collection). Other examples are checking
for exception error messages very explicitly. However, the CPython test suite
has gotten a lot better in these regards over time, by adding
support.gc_collect()
calls to fix the former problem, and by marking some
very specific tests with the @impl_detail
decorator. Thanks to all the
CPython developers who have worked on this!
In the process of re-implementing CPython's functionality and running CPython's tests suite, PyPy can often also be a good way to find bugs in CPython. While we think about the corner cases of some Python feature we occasionally find situations where CPython didn't get everything completely correct either, which we then report back.
Testing for Performance Regressions
All the tests we described so far are checking behaviour. But one of PyPy's important goals is to be a fast implementation not "just" a correct one. Some aspects of performance can be tested by regular unit tests, either application- or interpreter-level. In order to check whether some performance shortcut is taken in the interpreter, we sometimes can write tests that monkeypatch the slow default implementation to always error. Then, if the fast path is taken properly, that slow default implementation is never reached.
But we also have additional tests that test the correct interaction with the JIT explicitly. For that, we have a special style of test that checks that the JIT will produce the correct machine code for a small snippet of Python code. To make this kind of test somewhat more robust, we don't check the machine code directly, but instead the architecture independent intermediate representation that the JIT uses to produce machine code from.
As an example, here is a small test that loading the attribute of a constant global instance can be completely constant folded away:
def test_load_attr(self): src = ''' class A(object): pass a = A() a.x = 1 def main(n): i = 0 while i < n: i = i + a.x return i ''' log = self.run(src, [1000]) assert log.result == 1000 loop, = log.loops_by_filename(self.filepath) assert loop.match(""" i9 = int_lt(i5, i6) guard_true(i9, descr=...) guard_not_invalidated(descr=...) i10 = int_add(i5, 1) --TICK-- jump(..., descr=...) """)
The string passed to the loop.match
function is a string representation of
the intermediate representation code that is generated for the while
loop in
the main
function given in the source. The important part of that
intermediate representation is that the i = i + a.x
addition is optimized
into an int_add(x, 1)
operation. The second argument for the addition is the
constant 1
, because the JIT noted that the global a
is a constant, and
the attribute x
of that instance is always 1
. The test thus checks that
this optimization still works.
Those tests are again more on the unit test side of things (and can thus unfortunately be a bit brittle sometimes and break). The integration test equivalent for performance is the PyPy Speed Center which tracks the performance of micro- and macro-benchmarks over time and lets us see when big performance regressions are happening. The speed center is not really an automatic test and does not produce pass/fail outcomes. Instead, it requires human judgement and intervention in order to interpret the performance changes. Having a real pass/fail mechanism is something that would be great to have but is probably quite tricky in practice.
Conclusion
This concludes my overview of some of the different styles of tests that we use to develop the PyPy Python interpreter.
There is a whole other set of tests for the development of the RPython language, the garbage collectors it provides as well as the code that does the automatic JIT insertion, maybe I'll cover these in a future post.
Footnotes
¹ CPython has the _testcapimodule.c and related modules, that are used to
unit-test the C-API. However, these are still driven from Python tests using
the unittest
framework and wouldn't run without the Python interpreter
already working.
² There is also a deprecated different way to write these tests, by putting
them in the test_*.py
files that interpreter level tests are using and
then having a test class with the pattern class AppTest*
. We haven't
converted all of them to the new style yet, even though the old style is
quite weird: since the test_*.py
files are themselves parsed by
Python 2, the tests methods in AppTest*
classes need to be written in the
subset of Python 3 syntax that is also valid Python 2 syntax, leading to a lot
of confusion.
³ Nit-picky side-note: C interpreters are a thing! But not that widely used in practice, or only in very specific situations.
PyPy v7.3.9 security release
PyPy v7.3.9 security release
The PyPy team is proud to release version 7.3.9 of PyPy. This is a security
release to match the recent CPython release and updates the portable pypy
tarballs with bzip2 1.0.8
, openssl1.1.1n
, and libexpat 2.4.7
. Along
the way this release fixes some issues discovered after the 7.3.8 release and
updates sqlite3
to 3.38.2. It includes:
PyPy2.7, which is an interpreter supporting the syntax and the features of Python 2.7 including the stdlib for CPython 2.7.18+ (the
+
is for backported security updates)PyPy3.7, which is an interpreter supporting the syntax and the features of Python 3.7, including the stdlib for CPython 3.7.13. This will be the last release of PyPy3.7.
PyPy3.8, which is an interpreter supporting the syntax and the features of Python 3.8, including the stdlib for CPython 3.8.13.
PyPy3.9, which is an interpreter supporting the syntax and the features of Python 3.9, including the stdlib for CPython 3.9.12. We relate to this as "beta" quality. We welcome testing of this version, if you discover incompatibilities, please report them so we can gain confidence in the version.
The interpreters are based on much the same codebase, thus the multiple release. This is a micro release, all APIs are compatible with the other 7.3 releases. Highlights of the release, since the release of 7.3.8 in February 2022, include:
Fixed some failing stdlib tests on PyPy3.9
Update the bundled libexpat to 2.4.6 and sqlite3 to 3.38.2
We recommend updating. You can find links to download the v7.3.9 releases here:
We would like to thank our donors for the continued support of the PyPy project. If PyPy is not quite good enough for your needs, we are available for direct consulting work. If PyPy is helping you out, we would love to hear about it and encourage submissions to our blog via a pull request to https://github.com/pypy/pypy.org
We would also like to thank our contributors and encourage new people to join the project. PyPy has many layers and we need help with all of them: PyPy and RPython documentation improvements, tweaking popular modules to run on PyPy, or general help with making RPython's JIT even better. Since the 7.3.7 release, we have accepted contributions from 6 new contributors, thanks for pitching in, and welcome to the project!
If you are a python library maintainer and use C-extensions, please consider making a HPy / CFFI / cppyy version of your library that would be performant on PyPy. In any case both cibuildwheel and the multibuild system support building wheels for PyPy.
What is PyPy?
PyPy is a Python interpreter, a drop-in replacement for CPython 2.7, 3.7, 3.8 and 3.9. It's fast (PyPy and CPython 3.7.4 performance comparison) due to its integrated tracing JIT compiler.
We also welcome developers of other dynamic languages to see what RPython can do for them.
This PyPy release supports:
x86 machines on most common operating systems (Linux 32/64 bits, Mac OS X 64 bits, Windows 64 bits, OpenBSD, FreeBSD)
64-bit ARM machines running Linux. A shoutout to Huawei for sponsoring the VM running the tests.
s390x running Linux
big- and little-endian variants of PPC64 running Linux,
PyPy support Windows 32-bit, PPC64 big- and little-endian, and ARM 32 bit, but does not release binaries. Please reach out to us if you wish to sponsor releases for those platforms.
Known Issues with PyPy3.9
We slightly modified the concurrent future's
ProcessExcecutorPool
to start all the worker processes when the first task is received (like on Python3.8) to avoid an apparent race condition when usingfork
and threads (issue 3650).
What else is new?
For more information about the 7.3.9 release, see the full changelog.
Please update, and continue to help us make PyPy better.
Cheers, The PyPy team
PyPy v7.3.8: release of python 2.7, 3.7, 3.8, and 3.9
PyPy v7.3.8: release of python 2.7, 3.7, 3.8, and 3.9-beta
The PyPy team is proud to release version 7.3.8 of PyPy. It has been only a few months since our last release, but we have some nice speedups and bugfixes we wish to share. The release includes four different interpreters:
PyPy2.7, which is an interpreter supporting the syntax and the features of Python 2.7 including the stdlib for CPython 2.7.18+ (the
+
is for backported security updates)PyPy3.7, which is an interpreter supporting the syntax and the features of Python 3.7, including the stdlib for CPython 3.7.12. This will be the last release of PyPy3.7.
PyPy3.8, which is an interpreter supporting the syntax and the features of Python 3.8, including the stdlib for CPython 3.8.12. This is our third release of this interpreter, and we are removing the "beta" tag.
PyPy3.9, which is an interpreter supporting the syntax and the features of Python 3.9, including the stdlib for CPython 3.9.10. As this is our first release of this interpreter, we relate to this as "beta" quality. We welcome testing of this version, if you discover incompatibilities, please report them so we can gain confidence in the version.
The interpreters are based on much the same codebase, thus the multiple release. This is a micro release, all APIs are compatible with the other 7.3 releases. Highlights of the release, since the release of 7.3.7 in late October 2021, include:
PyPy3.9 uses an RPython version of the PEG parser which brought with it a cleanup of the lexer and parser in general
Fixed a regression in PyPy3.8 when JITting empty list comprehensions
Tweaked some issues around changing the file layout after packaging to make the on-disk layout of PyPy3.8 more compatible with CPython. This requires
setuptools>=58.1.0
RPython now allows the target executable to have a
.
in its name, so PyPy3.9 will produce apypy3.9-c
andlibpypy3.9-c.so
. Changing the name of the shared object to be version-specific (it used to belibpypy3-c.so
) will allow it to live alongside other versions.Building PyPy3.9+ accepts a
--platlibdir
argument like CPython.Improvement in ssl's use of CFFI buffers to speed up
recv
andrecvinto
Update the packaged OpenSSL to 1.1.1m
We recommend updating. You can find links to download the v7.3.8 releases here:
We would like to thank our donors for the continued support of the PyPy project. If PyPy is not quite good enough for your needs, we are available for direct consulting work. If PyPy is helping you out, we would love to hear about it and encourage submissions to our blog via a pull request to https://github.com/pypy/pypy.org
We would also like to thank our contributors and encourage new people to join the project. PyPy has many layers and we need help with all of them: PyPy and RPython documentation improvements, tweaking popular modules to run on PyPy, or general help with making RPython's JIT even better. Since the previous release, we have accepted contributions from 6 new contributors, thanks for pitching in, and welcome to the project!
If you are a python library maintainer and use C-extensions, please consider making a HPy / CFFI / cppyy version of your library that would be performant on PyPy. In any case both cibuildwheel and the multibuild system support building wheels for PyPy.
What is PyPy?
PyPy is a Python interpreter, a drop-in replacement for CPython 2.7, 3.7, 3.8 and 3.9. It's fast (PyPy and CPython 3.7.4 performance comparison) due to its integrated tracing JIT compiler.
We also welcome developers of other dynamic languages to see what RPython can do for them.
This PyPy release supports:
x86 machines on most common operating systems (Linux 32/64 bits, Mac OS X 64 bits, Windows 64 bits, OpenBSD, FreeBSD)
64-bit ARM machines running Linux. A shoutout to Huawei for sponsoring the VM running the tests.
s390x running Linux
big- and little-endian variants of PPC64 running Linux,
PyPy support Windows 32-bit, PPC64 big- and little-endian, and ARM 32 bit, but does not release binaries. Please reach out to us if you wish to sponsor releases for those platforms.
Known Issues with PyPy3.9
There is still a known speed regression around
**kwargs
handlingWe slightly modified the concurrent future's
ProcessExcecutorPool
to start all the worker processes when the first task is received (like on Python3.8) to avoid an apparent race condition when usingfork
and threads (issue 3650).
What else is new?
For more information about the 7.3.8 release, see the full changelog.
Please update, and continue to help us make PyPy better.
Cheers, The PyPy team
Natural Language Processing for Icelandic with PyPy: A Case Study
Natural Language Processing for Icelandic with PyPy: A Case Study
Icelandic is one of the smallest languages of the world, with about 370.000 speakers. It is a language in the Germanic family, most similar to Norwegian, Danish and Swedish, but closer to the original Old Norse spoken throughout Scandinavia until about the 14th century CE.
As with other small languages, there are worries that the language may not survive in a digital world, where all kinds of fancy applications are developed first - and perhaps only - for the major languages. Voice assistants, chatbots, spelling and grammar checking utilities, machine translation, etc., are increasingly becoming staples of our personal and professional lives, but if they don’t exist for Icelandic, Icelanders will gravitate towards English or other languages where such tools are readily available.
Iceland is a technology-savvy country, with world-leading adoption rates of the Internet, PCs and smart devices, and a thriving software industry. So the government figured that it would be worthwhile to fund a 5-year plan to build natural language processing (NLP) resources and other infrastructure for the Icelandic language. The project focuses on collecting data and developing open source software for a range of core applications, such as tokenization, vocabulary lookup, n-gram statistics, part-of-speech tagging, named entity recognition, spelling and grammar checking, neural language models and speech processing.
My name is Vilhjálmur Þorsteinsson, and I’m the founder and CEO of a software startup Miðeind in Reykjavík, Iceland, that employs 10 software engineers and linguists and focuses on NLP and AI for the Icelandic language. The company participates in the government’s language technology program, and has contributed significantly to the program’s core tools (e.g., a tokenizer and a parser), spelling and grammar checking modules, and a neural machine translation stack.
When it came to a choice of programming languages and development tools for the government program, the requirements were for a major, well supported, vendor-and-OS-agnostic FOSS platform with a large and diverse community, including in the NLP space. The decision to select Python as a foundational language for the project was a relatively easy one. That said, there was a bit of trepidation around the well known fact that CPython can be slow for inner-core tasks, such as tokenization and parsing, that can see heavy workloads in production.
I first became aware of PyPy in early 2016 when I was developing a crossword game Netskrafl in Python 2.7 for Google App Engine. I had a utility program that compressed a dictionary into a Directed Acyclic Word Graph and was taking 160 seconds to run on CPython 2.7, so I tried PyPy and to my amazement saw a 4x speedup (down to 38 seconds), with literally no effort besides downloading the PyPy runtime.
This led me to select PyPy as the default Python interpreter for my company’s Python development efforts as well as for our production websites and API servers, a role in which it remains to this day. We have followed PyPy’s upgrades along the way, being just about to migrate our minimally required language version from 3.6 to 3.7.
In NLP, speed and memory requirements can be quite important for software usability. On the other hand, NLP logic and algorithms are often complex and challenging to program, so programmer productivity and code clarity are also critical success factors. A pragmatic approach balances these factors, avoids premature optimization and seeks a careful compromise between maximal run-time efficiency and minimal programming and maintenance effort.
Turning to our use cases, our Icelandic text tokenizer "Tokenizer" is fairly light, runs tight loops and performs a large number of small, repetitive operations. It runs very well on PyPy’s JIT and has not required further optimization.
Our Icelandic parser Greynir (known on PyPI as reynir) is, if I may say so myself, a piece of work. It parses natural language text according to a hand-written context-free grammar, using an Earley-type algorithm as enhanced by Scott and Johnstone. The CFG contains almost 7,000 nonterminals and 6,000 terminals, and the parser handles ambiguity as well as left, right and middle recursion. It returns a packed parse forest for each input sentence, which is then pruned by a scoring heuristic down to a single best result tree.
This parser was originally coded in pure Python and turned out to be unusably slow when run on CPython - but usable on PyPy, where it was 3-4x faster. However, when we started applying it to heavier production workloads, it became apparent that it needed to be faster still. We then proceeded to convert the innermost Earley parsing loop from Python to tight C++ and to call it from PyPy via CFFI, with callbacks for token-terminal matching functions (“business logic”) that remained on the Python side. This made the parser much faster (on the order of 100x faster than the original on CPython) and quick enough for our production use cases. Even after moving much of the heavy processing to C++ and using CFFI, PyPy still gives a significant speed boost over CPython.
Connecting C++ code with PyPy proved to be quite painless using CFFI, although we had to figure out a few magic incantations in our build module to make it compile smoothly during setup from source on Windows and MacOS in addition to Linux. Of course, we build binary PyPy and CPython wheels for the most common targets so most users don’t have to worry about setup requirements.
With the positive experience from the parser project, we proceeded to take a similar approach for two other core NLP packages: our compressed vocabulary package BinPackage (known on PyPI as islenska) and our trigrams database package Icegrams. These packages both take large text input (3.1 million word forms with inflection data in the vocabulary case; 100 million tokens in the trigrams case) and compress it into packed binary structures. These structures are then memory-mapped at run-time using mmap and queried via Python functions with a lookup time in the microseconds range. The low-level data structure navigation is done in C++, called from Python via CFFI. The ex-ante preparation, packing, bit-fiddling and data structure generation is fast enough with PyPy, so we haven’t seen a need to optimize that part further.
To showcase our tools, we host public (and open source) websites such as greynir.is for our parsing, named entity recognition and query stack and yfirlestur.is for our spell and grammar checking stack. The server code on these sites is all Python running on PyPy using Flask, wrapped in gunicorn and hosted on nginx. The underlying database is PostgreSQL accessed via SQLAlchemy and psycopg2cffi. This setup has served us well for 6 years and counting, being fast, reliable and having helpful and supporting communities.
As can be inferred from the above, we are avid fans of PyPy and commensurately thankful for the great work by the PyPy team over the years. PyPy has enabled us to use Python for a larger part of our toolset than CPython alone would have supported, and its smooth integration with C/C++ through CFFI has helped us attain a better tradeoff between performance and programmer productivity in our projects. We wish for PyPy a great and bright future and also look forward to exciting related developments on the horizon, such as HPy.